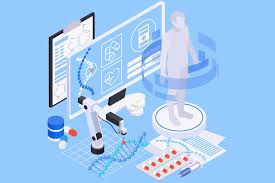
Introduction
Introduction to the significance of regulatory affairs in the pharmaceutical industry :-
Regulatory affairs play a crucial role in the pharmaceutical industry, ensuring that drugs are developed, tested, and brought to market in compliance with stringent safety, efficacy, and quality standards. This field involves navigating complex regulatory frameworks set by government agencies such as the FDA, EMA, and others, to ensure that pharmaceutical products meet legal and ethical requirements before reaching consumers. With the increasing complexity of global markets and evolving regulatory guidelines, the need for innovation in regulatory processes has never been greater. One of the most promising advancements is the application of AI in drug regulation, which is revolutionizing the way regulatory affairs professionals manage tasks such as drug approval, compliance monitoring, and post-market surveillance. By leveraging artificial intelligence, regulatory teams can streamline workflows, predict regulatory outcomes, and enhance decision-making, ultimately accelerating the development and approval of life-saving therapies while maintaining rigorous safety standards. As AI continues to evolve, its role in drug regulation is expected to expand, reshaping the future of regulatory affairs in the pharmaceutical industry.
Overview of how AI is becoming a central figure in reshaping drug regulation processes :-
Artificial intelligence is rapidly becoming a central figure in reshaping drug regulation processes, transforming how regulatory affairs professionals manage the approval and oversight of pharmaceutical products. Traditionally, regulatory processes have been time-consuming and resource-intensive, with complex manual reviews of clinical data, safety reports, and compliance documentation. However, the integration of AI in drug regulation is streamlining these procedures, offering powerful tools to analyze vast amounts of data more quickly and accurately. AI algorithms can assist in predicting drug approval outcomes, identifying potential regulatory issues before they arise, and ensuring that clinical trials meet compliance standards in real-time. Additionally, machine learning models are being used to monitor post-market safety and efficacy, enabling faster detection of adverse events and more proactive risk management. By enhancing decision-making and automating repetitive tasks, AI is not only increasing the efficiency of regulatory processes but also improving the accuracy and reliability of drug evaluations. As AI continues to evolve, its influence is expected to grow, paving the way for a more dynamic, data-driven regulatory landscape that can keep pace with the rapid advancements in pharmaceutical science.
Kick off your course with Company Connect Consultancy by following this link: https://shorturl.at/LVq6n
Understanding Regulatory Affairs
Explanation of what regulatory affairs entail in the context of pharmaceuticals :-
Regulatory affairs in the pharmaceutical industry encompass a broad range of activities aimed at ensuring that drugs and medical devices meet the required legal, safety, and efficacy standards before they are marketed and made available to the public. This involves navigating complex regulatory frameworks set by national and international health authorities, such as the FDA, EMA, and WHO, throughout a product’s lifecycle—from development through to post-market surveillance. Regulatory affairs professionals are responsible for preparing and submitting documentation to regulatory bodies, overseeing clinical trial compliance, ensuring labeling and advertising conform to regulations, and managing audits and inspections. In recent years, the integration of AI in drug regulation has introduced innovative ways to streamline these tasks. AI technologies help regulatory teams automate the analysis of vast datasets, improve the accuracy of regulatory submissions, and predict approval outcomes with greater speed. AI also enhances the monitoring of drug safety post-market by analyzing real-world data for signs of adverse events, ensuring ongoing compliance with evolving regulations. As regulatory affairs continue to evolve, the incorporation of AI is expected to further enhance efficiency, reduce human error, and accelerate the drug approval process.
Importance of regulatory compliance to ensure safety and efficacy of drugs :-
Regulatory compliance is of paramount importance in the pharmaceutical industry, as it ensures that drugs meet the highest standards of safety, efficacy, and quality before they reach patients. Regulatory agencies, such as the FDA and EMA, set rigorous guidelines to protect public health, requiring extensive clinical testing, data analysis, and ongoing monitoring to confirm that drugs are both safe and effective for their intended use. Compliance with these regulations is critical to prevent harmful side effects, improve patient outcomes, and ensure that treatments perform as promised. In this context, AI in drug regulation plays a transformative role by streamlining the compliance process. AI tools can rapidly analyze clinical data, flagging potential safety risks or inconsistencies early in the development cycle, helping companies adhere to regulatory standards more effectively. Additionally, machine learning models can predict regulatory outcomes, ensuring faster and more accurate submissions. AI-powered analytics are also increasingly used in post-market surveillance to track real-world drug performance, identify adverse events, and ensure continuous compliance with evolving regulations. Ultimately, the integration of AI enhances the ability to maintain high standards of regulatory compliance, safeguarding both public health and the integrity of the pharmaceutical industry.
Kick off your course with Company Connect Consultancy by following this link: https://shorturl.at/LVq6n
Introduction of AI in Drug Regulation
Brief history of AI applications in various industries :-
The history of AI applications spans several decades, with early developments in the 1950s focused on theoretical concepts such as machine learning and neural networks. Over the years, AI has evolved from a research curiosity into a transformative force across numerous industries. In manufacturing, AI has been used to optimize production lines and enhance supply chain management, while in finance, it has revolutionized fraud detection, trading algorithms, and customer service. The healthcare sector has seen AI applications in diagnostics, personalized medicine, and predictive analytics. One of the most promising and rapidly growing areas of AI integration is in the field of AI in drug regulation. In the pharmaceutical industry, AI has begun to reshape how regulatory compliance is achieved, making it easier to navigate complex regulatory environments and speeding up the drug approval process. Machine learning algorithms can analyze vast datasets from clinical trials, predict regulatory outcomes, and automate documentation processes, which are critical for meeting the stringent requirements set by regulatory bodies. As AI technologies continue to evolve, their influence is increasingly felt in drug regulation, offering the potential to accelerate the development of safe and effective medications while maintaining high standards of compliance and safety.
Transition of AI into the field of regulatory affairs and its implications :-
The transition of AI into the field of regulatory affairs marks a significant shift in how pharmaceutical companies approach drug development, approval, and compliance. Traditionally, regulatory affairs have been characterized by complex, manual processes that involve extensive documentation, data analysis, and interactions with regulatory bodies. However, with the rise of AI in drug regulation, these processes are becoming more efficient and data-driven. Machine learning algorithms, natural language processing, and predictive analytics are now being used to analyze clinical trial data, streamline regulatory submissions, and assess drug safety in real-time. The implications of this transition are profound, as AI can significantly shorten the timelines for drug approval, reduce human error in data analysis, and enhance the accuracy of regulatory filings. Additionally, AI's ability to monitor post-market data helps identify potential safety issues more quickly, enabling faster responses to emerging risks. This integration of AI not only increases the efficiency of regulatory affairs but also helps ensure that the drugs that reach the market meet the highest standards of safety and efficacy. As AI continues to evolve, its role in drug regulation will likely expand, leading to more agile, responsive, and data-centric regulatory environments across the pharmaceutical industry.
Kick off your course with Company Connect Consultancy by following this link: https://shorturl.at/LVq6n
Key Roles of AI in Regulatory Affairs
Data Analysis and Management:
AI’s capabilities in processing vast amounts of data for regulatory submissions :-
One of the most significant advantages of AI in the pharmaceutical industry is its ability to process vast amounts of data quickly and accurately, particularly in the context of regulatory submissions. Traditionally, compiling and reviewing the extensive documentation required for drug approvals, including clinical trial data, safety reports, and efficacy results, was a labor-intensive and time-consuming process. However, with the integration of AI in drug regulation, this process is becoming much more streamlined and efficient. AI algorithms can analyze large datasets in real time, identifying patterns, inconsistencies, or potential issues that may not be immediately obvious to human reviewers. For example, AI tools can automatically flag regulatory inconsistencies, cross-check data across multiple sources, and optimize the format and structure of submissions to meet specific regulatory requirements. This capability not only speeds up the submission process but also reduces the likelihood of errors, ensuring that all necessary information is accurately presented to regulatory agencies. Additionally, AI can predict the likelihood of approval based on historical data and regulatory trends, providing valuable insights to regulatory affairs teams and helping them better navigate complex approval pathways. As AI continues to advance, its ability to handle large-scale data processing will become an even more integral part of AI in drug regulation, accelerating drug development and bringing safe, effective treatments to market faster.
Enhanced accuracy in data analysis compared to traditional methods :-
One of the key advantages of AI in drug regulation is its enhanced accuracy in data analysis, which significantly surpasses traditional manual methods. Regulatory submissions often involve analyzing complex datasets, including clinical trial results, adverse event reports, and compliance documentation. In conventional approaches, this process is prone to human error due to the sheer volume of data and the intricate nature of regulatory requirements. AI, however, utilizes advanced algorithms and machine learning models to systematically process and analyze vast amounts of data with far greater precision. For example, AI can identify subtle patterns or inconsistencies in clinical trial data that might be overlooked by human analysts, ensuring that all critical safety and efficacy information is captured accurately. Additionally, AI systems can cross-reference data from multiple sources, verifying the integrity and consistency of the information, and highlighting potential discrepancies that could delay approval. This level of accuracy not only minimizes the risk of errors but also ensures that regulatory submissions are more thorough and compliant with stringent standards. As AI continues to advance, its ability to enhance data analysis in AI in drug regulation will further streamline the approval process, leading to faster, more reliable drug assessments and ultimately improving public health outcomes.
Risk Assessment:
The utilization of AI algorithms to predict potential risks involved in drug designs :-
The utilization of AI algorithms to predict potential risks in drug designs represents a major advancement in AI in drug regulation, significantly improving both the efficiency and safety of drug development. Traditional drug design often involves a lengthy process of trial and error, where researchers rely on experience and extensive laboratory testing to assess the safety and efficacy of new compounds. However, AI can analyze vast datasets of historical research, clinical trial outcomes, and real-world evidence to identify patterns and predict potential risks much earlier in the development process. For example, AI models can assess a drug's molecular structure and predict how it may interact with biological systems, helping to identify possible side effects or toxicity risks before clinical trials begin. Additionally, machine learning algorithms can predict how environmental factors or patient demographics might influence a drug's performance, further enhancing risk management. By providing a data-driven, proactive approach to risk assessment, AI in drug regulation helps regulatory agencies and pharmaceutical companies make more informed decisions, reducing the likelihood of costly delays or safety issues in later stages of development. As AI continues to evolve, its predictive capabilities are expected to play an even greater role in identifying potential risks early, ultimately ensuring safer, more effective drugs are brought to market.
Improvement in risk management strategies through predictive analytics :-
The improvement in risk management strategies through predictive analytics is one of the most transformative aspects of AI in drug regulation. Traditional risk management in drug development often relied on reactive measures, where safety concerns were addressed only after clinical trials or post-market surveillance. However, with the power of AI, pharmaceutical companies and regulatory bodies can now take a more proactive approach. AI-driven predictive analytics can analyze vast datasets from clinical trials, preclinical studies, and real-world evidence to identify potential risks early in the drug development process. By recognizing patterns in data that may indicate safety concerns—such as adverse drug reactions or interactions—AI models can forecast potential issues before they arise in clinical settings. These predictive insights allow for more informed decision-making, enabling developers to adjust drug formulations or trial designs to mitigate risks before they become significant problems. Moreover, AI in drug regulation can enhance post-market risk management by continuously analyzing real-time data from patient populations, identifying emerging risks, and facilitating quicker regulatory responses. Ultimately, AI’s predictive capabilities are revolutionizing how risks are managed throughout the lifecycle of a drug, improving both safety and compliance, and leading to more efficient regulatory processes.
Communication Streamlining:
AI chatbots and virtual assistants improving communication between regulatory bodies and pharmaceutical companies :-
AI chatbots and virtual assistants are rapidly transforming communication between regulatory bodies and pharmaceutical companies, playing an increasingly vital role in AI in drug regulation. Traditionally, regulatory interactions—such as clarifications on submission requirements, compliance queries, and approval status updates—were often time-consuming, relying on email exchanges or phone calls that could delay decision-making. With the advent of AI-powered chatbots and virtual assistants, this communication has become much more efficient and streamlined. These AI tools can provide instant, automated responses to frequently asked questions, guiding pharmaceutical companies through complex regulatory requirements, helping them prepare accurate submissions, and offering updates on regulatory processes in real time. By leveraging natural language processing, AI chatbots can understand and respond to intricate regulatory queries, offering contextually relevant advice and reducing the workload for human regulatory officers. Furthermore, these AI-driven solutions can operate 24/7, ensuring continuous support for pharmaceutical companies across different time zones. The enhanced responsiveness and efficiency of AI in facilitating communication not only accelerates the regulatory process but also ensures a smoother collaboration between pharmaceutical companies and regulatory agencies, leading to faster approvals and better adherence to compliance standards.
Reduction in delays due to more efficient inquiry handling :-
One of the key benefits of AI in drug regulation is the reduction in delays through more efficient inquiry handling between pharmaceutical companies and regulatory bodies. In traditional regulatory processes, the time required to address queries, clarify submission requirements, or resolve compliance issues could lead to significant delays in the drug approval process. These inquiries often involved manual reviews, back-and-forth communications, and waiting for responses from regulatory authorities, all of which could extend timelines and hinder the development of life-saving therapies. However, with the integration of AI-powered systems, this process has become much more streamlined. AI-driven chatbots and virtual assistants can quickly handle routine inquiries, providing instant responses to frequently asked questions, clarifying regulatory requirements, and offering guidance on submission statuses. This automation not only accelerates communication but also ensures that human resources can focus on more complex tasks that require expert input. As a result, regulatory inquiries are resolved much faster, minimizing unnecessary delays and helping pharmaceutical companies stay on track with their development timelines. By enabling quicker decision-making and response times, AI in drug regulation is significantly improving the efficiency of the drug approval process, ultimately bringing safe and effective drugs to market more rapidly.
Kick off your course with Company Connect Consultancy by following this link: https://shorturl.at/LVq6n
AI and Clinical Trials
Patient Recruitment and Data Collection:
AI’s role in identifying suitable patient populations for clinical trials :-
AI plays a crucial role in identifying suitable patient populations for clinical trials, significantly enhancing the precision and efficiency of clinical trial design in the context of AI in drug regulation. One of the most challenging aspects of clinical trials is selecting the right patient cohort that accurately represents the target population, while ensuring safety and efficacy across different demographics. Traditionally, this selection process was largely manual, relying on patient records, physician expertise, and general inclusion/exclusion criteria, which could be time-consuming and prone to bias. However, AI algorithms can now analyze vast amounts of patient data, including medical histories, genetic information, and even lifestyle factors, to identify individuals who meet the specific criteria for a given clinical trial. Machine learning models can uncover hidden patterns in this data, allowing for more precise segmentation of patient populations based on factors like genetic markers, comorbidities, or prior treatment responses. This not only improves the likelihood of trial success but also helps regulatory bodies ensure that trials reflect diverse patient needs and safety standards. As AI in drug regulation continues to evolve, its ability to optimize patient selection will further streamline the clinical trial process, reducing recruitment time, improving trial outcomes, and accelerating the overall drug development timeline.
Automation of data collection processes enhancing trial efficiency :-
Automation of data collection processes through AI is revolutionizing the efficiency of clinical trials, a critical aspect of AI in drug regulation. In traditional clinical trials, data collection is often a labor-intensive and error-prone process, requiring extensive manual entry, patient monitoring, and paperwork. This can lead to delays, inaccuracies, and increased costs, ultimately slowing down the drug development process. However, AI-driven automation tools are streamlining this process by digitizing and integrating data collection, allowing for real-time updates and reducing the need for manual intervention. For instance, AI can automatically capture patient data from electronic health records (EHRs), wearable devices, or mobile health apps, ensuring accurate and continuous monitoring of patient conditions. This not only accelerates data acquisition but also improves the consistency and reliability of the data being collected. By automating routine data entry, AI frees up clinical staff to focus on higher-value tasks, such as patient care and decision-making. Furthermore, AI can analyze data on-the-fly, providing insights into trial progress and identifying potential issues early, such as data inconsistencies or safety concerns. As a result, AI in drug regulation is enhancing the overall efficiency of clinical trials, enabling faster, more accurate data collection, and ultimately leading to quicker, more reliable regulatory submissions and drug approvals.
Real-time Monitoring:
AI systems designed to monitor trial data in real-time for safety concerns :-
AI systems designed to monitor trial data in real-time are playing a pivotal role in enhancing the safety and oversight of clinical trials, a key component of AI in drug regulation. Traditionally, safety monitoring during clinical trials has involved periodic data reviews and manual reporting, which could lead to delays in identifying adverse events or other safety concerns. However, with the integration of AI, real-time monitoring has become more proactive and efficient. AI-powered systems can continuously analyze data from various sources—such as patient health records, biometric devices, and lab results—detecting patterns or anomalies that might indicate emerging safety issues, such as unexpected side effects or adverse reactions. These systems can flag potential concerns immediately, allowing clinical teams and regulatory bodies to respond more quickly, adjust trial protocols, or even halt trials if necessary to protect patient safety. The ability to monitor vast amounts of data in real time significantly enhances the early detection of safety risks, ensuring that regulatory standards are maintained throughout the trial process. As AI in drug regulation continues to evolve, the use of AI for real-time safety monitoring will further strengthen patient protection and improve the overall integrity of clinical trials, enabling faster and more reliable decision-making.
Early identification of adverse effects escalating response time to safeguard participants :-
The early identification of adverse effects through AI in drug regulation is transforming how clinical trials respond to potential safety concerns, significantly improving response times and safeguarding participants. In traditional clinical trials, detecting adverse effects often relied on periodic data reviews and patient reports, which could delay appropriate actions and put participants at risk. However, AI systems can now continuously monitor vast amounts of trial data in real-time, including biometric readings, lab results, and patient feedback, to quickly identify any signs of adverse reactions. By analyzing patterns in this data, AI can detect subtle, early warning signals of side effects that may otherwise go unnoticed. When potential issues are flagged, clinical teams and regulatory bodies can act swiftly to assess the severity of the risk, modify trial protocols, or even suspend a trial to protect patient safety. This capability not only enhances patient protection but also helps ensure compliance with regulatory requirements. The speed at which AI can escalate responses to safety concerns minimizes the chances of serious harm to participants, demonstrating the vital role of AI in drug regulation in improving the overall integrity and safety of clinical trials. As AI continues to advance, its ability to identify risks early will further accelerate decision-making, leading to safer and more efficient drug development.
Kick off your course with Company Connect Consultancy by following this link: https://shorturl.at/LVq6n
Predictive Analytics in Drug Evaluation
Explanation of how AI predicts drug performance based on historical data :-
AI’s ability to predict drug performance based on historical data is one of its most powerful applications in AI in drug regulation, helping pharmaceutical companies and regulatory bodies make more informed decisions during the drug development process. By analyzing large volumes of historical clinical trial data, patient outcomes, and even data from preclinical studies, AI algorithms can uncover patterns and correlations that are not immediately apparent to human researchers. For example, machine learning models can assess how similar drugs have performed across different populations, predict how a new drug might interact with specific patient demographics, or forecast the likelihood of adverse events based on historical safety profiles. These predictions can provide valuable insights into a drug’s potential efficacy and safety before it enters the clinical trial phase, enabling developers to fine-tune their formulations or trial designs accordingly. Additionally, AI can predict the likelihood of regulatory approval by comparing the performance of a drug to previous approvals in similar therapeutic areas, helping companies navigate the complex regulatory landscape. By leveraging AI in drug regulation, pharmaceutical companies can make data-driven decisions, reduce the risk of failure, and accelerate the path to market, all while maintaining high standards of safety and compliance.
Introduction to machine learning models used in determining drug approval likelihood :-
Machine learning models have become a crucial tool in determining the likelihood of drug approval, significantly enhancing the efficiency and accuracy of the regulatory process, a key component of AI in drug regulation. These models are trained on vast datasets that include historical clinical trial results, regulatory submissions, approval histories, and real-world data, allowing them to identify patterns and trends that may predict the success or failure of a drug’s approval. By analyzing factors such as the drug’s efficacy in preclinical studies, safety profiles from clinical trials, and comparisons with similar drugs already on the market, machine learning algorithms can estimate the probability of approval by regulatory agencies like the FDA or EMA. These models can also consider variables like market conditions, regulatory shifts, and patient demographics, which further refine their predictions. This predictive capability allows pharmaceutical companies to make data-driven decisions about which drugs to prioritize, adjust development strategies, or modify clinical trial designs to better align with regulatory expectations. AI in drug regulation, powered by machine learning, is transforming how drug developers and regulators approach the approval process, reducing uncertainty and speeding up the time it takes to bring safe, effective drugs to market.
Kick off your course with Company Connect Consultancy by following this link: https://shorturl.at/LVq6n
Benefits of Integrating AI into Regulatory Processes
Increased Efficiency:
Reduction in the time required for regulatory submissions and approvals :-
One of the most significant benefits of AI in drug regulation is the reduction in the time required for regulatory submissions and approvals. Traditionally, the process of preparing and submitting regulatory filings, including clinical trial data, safety reports, and compliance documentation, has been lengthy and complex, often taking several months or even years. This delay is due to the extensive manual effort involved in gathering, verifying, and formatting data to meet the specific requirements of regulatory agencies. However, AI technologies are transforming this process by automating data collection, analysis, and submission preparation. AI-driven tools can quickly organize vast amounts of data, ensuring that all required information is accurate and compliant with regulatory standards. Additionally, AI models can predict potential regulatory hurdles early, enabling pharmaceutical companies to address issues before they arise, which helps avoid lengthy back-and-forth with regulatory bodies. By streamlining the submission process, automating routine tasks, and identifying potential issues proactively, AI in drug regulation significantly shortens approval timelines, accelerating the delivery of life-saving therapies to market while maintaining rigorous safety and efficacy standards.
Greater throughput in processing applications and addressing queries :-
AI in drug regulation is driving greater throughput in processing applications and addressing queries, significantly improving the efficiency of regulatory affairs. Traditionally, regulatory agencies and pharmaceutical companies have faced challenges in managing the volume of applications, submissions, and inquiries, often leading to delays in drug approvals and a backlog of unanswered questions. However, with the integration of AI, the entire process has become much more streamlined. AI-powered systems can quickly process large volumes of applications, automating the extraction and validation of critical data, ensuring that submissions meet all regulatory requirements in a fraction of the time it would take manually. Similarly, AI-driven chatbots and virtual assistants are now capable of instantly responding to routine queries from pharmaceutical companies, providing real-time updates on submission statuses, clarifying regulatory guidelines, and addressing compliance-related questions. By automating these repetitive tasks, AI reduces the workload on human regulators, allowing them to focus on more complex and critical issues. This increased throughput not only accelerates the approval process but also improves overall communication between pharmaceutical companies and regulatory bodies, leading to faster responses and more efficient decision-making across the board. As AI in drug regulation continues to evolve, its capacity to handle a greater volume of applications and queries will continue to optimize the drug approval lifecycle, benefiting both regulators and the pharmaceutical industry.
Cost-Effectiveness:
Lower operational costs due to reduced manual labor and faster processing times :-
One of the key advantages of AI in drug regulation is its potential to lower operational costs by significantly reducing the need for manual labor and accelerating processing times. In traditional regulatory processes, considerable resources are spent on manually reviewing applications, verifying data, and responding to queries from pharmaceutical companies. This not only requires a large workforce but also leads to longer turnaround times, increasing operational costs. However, with the integration of AI, many of these tasks can be automated. AI systems can efficiently handle data extraction, validation, and analysis, ensuring that submissions meet regulatory standards without the need for extensive human intervention. Additionally, AI-driven tools can quickly identify discrepancies or potential issues in regulatory filings, reducing the time spent on revisions and re-submissions. The use of AI-powered chatbots and virtual assistants also streamlines communication by providing instant responses to routine inquiries, further reducing the need for manual labor. With faster processing times and less reliance on human resources, pharmaceutical companies and regulatory bodies alike can realize significant cost savings, while improving efficiency and speed in drug approvals. As AI in drug regulation continues to mature, its ability to reduce operational costs and enhance productivity will be increasingly invaluable in an industry where time and resources are critical.
Financial savings in lengthy traditional review processes :-
The integration of AI in drug regulation offers significant financial savings by reducing the inefficiencies associated with lengthy traditional review processes. In the past, regulatory reviews required considerable time and resources, with human reviewers manually scrutinizing vast amounts of data, clinical trial results, and compliance documentation. These processes were not only time-consuming but also costly, as they involved extensive labor, administrative overhead, and delays in decision-making. However, AI technologies are transforming this landscape by automating many of these tasks, significantly cutting down on the time spent on data analysis, document preparation, and error correction. AI algorithms can quickly identify key information, cross-check data for inconsistencies, and flag potential issues early in the submission process, reducing the need for multiple rounds of revisions. This automation not only accelerates the review cycle but also minimizes the risk of errors, which could lead to costly delays or regulatory setbacks. By streamlining workflows, enhancing accuracy, and speeding up decision-making, AI in drug regulation helps pharmaceutical companies and regulatory bodies save both time and money, ultimately lowering operational costs and accelerating the delivery of drugs to market. As AI continues to evolve, its role in reducing financial inefficiencies will only grow, providing even greater cost savings for the industry.
Enhanced Compliance:
Improvement of compliance checks through automated systems minimizing human error :-
One of the most impactful ways AI in drug regulation is improving compliance is through the automation of regulatory checks, which minimizes human error and ensures greater accuracy throughout the drug approval process. In traditional regulatory workflows, compliance checks often involve labor-intensive manual reviews of vast amounts of data, clinical trial reports, and submission documents. This process is prone to human error, with the potential for overlooked details, inconsistencies, or missed regulatory requirements that could delay approvals or result in costly corrections. AI, however, can automate these compliance checks, instantly scanning submissions for completeness, consistency, and adherence to specific regulatory standards. AI-powered systems can cross-reference data with regulatory databases, flag discrepancies, and ensure that all necessary documentation is included before submission, greatly reducing the risk of errors. By identifying potential compliance issues early in the process, AI not only accelerates the approval timeline but also enhances the overall quality of submissions. This level of automation helps regulatory agencies and pharmaceutical companies maintain high standards of safety, efficacy, and regulatory adherence, while significantly reducing the chances of costly mistakes. As AI in drug regulation continues to evolve, the role of automated compliance checks will become increasingly critical in maintaining the integrity of the regulatory process and ensuring the safe and efficient approval of new drugs.
Continuous monitoring capabilities ensuring ongoing adherence to regulatory standards :-
AI in drug regulation plays a pivotal role in ensuring ongoing adherence to regulatory standards through continuous monitoring capabilities, which enhance both pre- and post-market surveillance. While traditional regulatory processes focus largely on the approval phase, AI allows for real-time, continuous monitoring of a drug’s performance and compliance throughout its lifecycle. AI systems can track vast amounts of data from clinical trials, real-world patient outcomes, and post-market surveillance to ensure that drugs remain compliant with regulatory standards even after reaching the market. For instance, AI can monitor adverse event reports, evaluate patient safety data, and assess the long-term efficacy of a drug, flagging potential issues as they arise. By utilizing machine learning models to analyze trends and detect anomalies, AI can identify deviations from regulatory requirements—such as new safety concerns or improper labeling—prompting quicker corrective actions. This level of continuous oversight ensures that pharmaceutical companies stay proactive in addressing any compliance issues, mitigating risks, and maintaining patient safety. Furthermore, AI in drug regulation can automate the generation of compliance reports and streamline communication with regulatory agencies, ensuring that all necessary updates are provided in a timely and accurate manner. As AI continues to advance, its ability to provide continuous, real-time monitoring will be essential for ensuring that drugs not only meet initial regulatory requirements but also remain in full compliance as they are used by broader patient populations.
Kick off your course with Company Connect Consultancy by following this link: https://shorturl.at/LVq6n
Future Outlook
Predictions on how AI will evolve within regulatory affairs :-
As AI in drug regulation continues to evolve, its impact on regulatory affairs is expected to grow exponentially, transforming how the pharmaceutical industry navigates compliance, approval processes, and post-market surveillance. In the near future, AI will likely become even more integrated into every stage of the drug development lifecycle, from early drug discovery to long-term monitoring of market performance. Machine learning models will continue to refine their ability to predict the likelihood of drug approval, helping pharmaceutical companies streamline their submissions and optimize clinical trial designs based on data-driven insights. AI-powered tools will become even more adept at handling complex regulatory submissions by automating more sophisticated tasks, such as identifying subtle safety risks, forecasting long-term drug interactions, and analyzing diverse patient populations for trial suitability. In addition, AI in drug regulation is poised to enhance global regulatory harmonization by enabling better data sharing and collaboration across borders. AI systems could facilitate real-time tracking of drug safety across international markets, quickly detecting adverse events and ensuring swift regulatory responses. Furthermore, as AI technology improves, we may see the development of "smart" regulatory systems that not only identify compliance issues but autonomously make recommendations or decisions based on evolving regulations and market conditions. Ultimately, the future of AI in drug regulation holds great promise, offering the potential for faster, safer, and more efficient drug approval processes, while maintaining the high standards required to protect public health.
The potential for AI to revolutionize drug discovery, safety monitoring, and compliance verification :-
The potential for AI in drug regulation to revolutionize drug discovery, safety monitoring, and compliance verification is vast and transformative. In drug discovery, AI can accelerate the identification of promising drug candidates by analyzing vast datasets, including genetic information, molecular structures, and clinical trial results, to predict how new compounds will interact with biological systems. This allows for more efficient screening of potential therapies and can significantly reduce the time and cost traditionally associated with the early stages of drug development. In terms of safety monitoring, AI's ability to process real-time data from clinical trials, electronic health records, and post-market surveillance enables continuous, proactive detection of adverse events. AI systems can flag potential safety risks before they escalate, helping regulators and pharmaceutical companies respond quickly to emerging issues and ensuring ongoing patient safety. Furthermore, AI is enhancing compliance verification by automating the analysis of regulatory submissions, ensuring that all necessary data, documentation, and safety information are included, and that they meet regulatory standards. With the capacity to continuously monitor drug performance and flag any discrepancies in real-time, AI can dramatically reduce human error, improve compliance, and ensure that drugs remain in adherence with evolving regulatory requirements. The combined impact of AI on these critical aspects of drug regulation is poised to not only speed up the development and approval of life-saving therapies but also enhance the safety and compliance of drugs throughout their lifecycle.
Kick off your course with Company Connect Consultancy by following this link: https://shorturl.at/LVq6n
Conclusion
Artificial intelligence (AI) is poised to have a transformative impact on regulatory affairs within the pharmaceutical industry, revolutionizing processes across the entire drug lifecycle, from discovery to post-market surveillance, while simultaneously improving the efficiency, accuracy, and responsiveness of regulatory frameworks. The ability of AI to analyze vast and complex datasets at unprecedented speeds offers immense potential to optimize regulatory approval timelines, streamline compliance checks, enhance safety monitoring, and predict drug performance with far greater precision than traditional methods. One of the most significant contributions of AI is its role in accelerating drug discovery, where AI-powered algorithms can sift through massive datasets, identify novel drug targets, and predict drug efficacy and toxicity early in the development process. By leveraging historical data, AI enables researchers to prioritize the most promising compounds, reducing the time, cost, and high failure rates associated with traditional drug development pipelines. Furthermore, AI's ability to integrate multi-modal data, such as genomic information, clinical trial outcomes, and real-world evidence, allows for more personalized medicine, helping to design drugs tailored to specific patient populations, and predicting adverse drug reactions based on genetic factors. In clinical trials, AI enhances patient recruitment by identifying suitable candidates based on complex eligibility criteria and minimizing recruitment biases, ensuring diverse representation in trials and optimizing recruitment efficiency. Real-time monitoring powered by AI also offers the ability to detect safety signals earlier in clinical trials and post-market, enabling faster response times to emerging safety concerns, potentially preventing severe adverse events and improving patient outcomes. Additionally, AI’s role in regulatory compliance is revolutionizing how pharmaceutical companies interact with regulatory agencies, automating the preparation of regulatory submissions, continuously ensuring adherence to evolving guidelines, and providing real-time tracking of compliance status across global markets. The potential of AI to automate document management, audit trails, and real-time data verification significantly reduces human error and resource-intensive manual oversight, speeding up approval processes and enabling more accurate, comprehensive filings. With continuous monitoring capabilities, AI-driven systems can now track ongoing drug production, clinical trial conduct, and post-market activities, ensuring that companies remain in compliance with regulatory standards even after a drug reaches the market, thus fostering a more transparent, responsive, and efficient regulatory environment. However, as AI continues to evolve, a balance must be struck between innovation and regulatory oversight to ensure that patient safety is always prioritized. While AI’s efficiency and predictive capabilities present unparalleled opportunities to speed up drug development and regulatory approvals, it also raises critical questions about the need for maintaining rigorous safety protocols and human oversight. One key challenge is ensuring that AI models themselves are transparent, interpretable, and reliable, as the complexity of machine learning algorithms can sometimes make their decision-making processes difficult to understand, raising concerns about accountability and trust. Furthermore, as AI systems become more integral to regulatory decision-making, there must be a continued focus on the ethical implications of AI applications in healthcare. Regulatory bodies must ensure that AI is not only designed to optimize speed and efficiency but also incorporates safeguards to mitigate risks, including biases in data or algorithms, and ensures equitable access to new treatments. Comprehensive guidelines will be necessary to ensure that AI-driven processes complement human expertise and do not replace it entirely, maintaining the critical role of human judgment in assessing risks, especially when it comes to the safety and well-being of patients. In maintaining this balance, regulatory agencies must also evolve in tandem with technological advancements, fostering an agile regulatory framework that can quickly adapt to the new realities of AI-powered drug development while upholding the high standards for patient safety that have long defined the industry. As AI systems become increasingly sophisticated, regulators will need to establish clear standards for validating AI models, monitoring their performance over time, and addressing any potential issues that arise. This includes ensuring that AI systems are continually trained on the most up-to-date data, and that potential risks, such as unforeseen side effects or interactions, are promptly identified and addressed. Furthermore, international collaboration will be key in harmonizing AI-driven regulatory frameworks, ensuring that AI tools can be used consistently across borders, particularly as the globalization of drug development continues. The ability to integrate AI into regulatory affairs at a global scale holds the potential to reduce inefficiencies, streamline approvals, and enhance the transparency and reliability of regulatory processes worldwide. Ultimately, the successful integration of AI into regulatory affairs hinges on a careful balance between embracing technological innovation and preserving the principles of caution, transparency, and patient-centered care. While AI promises to make the drug development and approval process faster, cheaper, and more effective, it must always be employed with an unwavering commitment to patient safety, ethical standards, and regulatory integrity. In this evolving landscape, both AI and regulatory oversight will need to complement each other, ensuring that new drugs and therapies are not only cutting-edge but also safe, effective, and equitable for all patients. This collaborative approach will unlock the full potential of AI while safeguarding the public trust in pharmaceutical innovation and regulatory processes alike.
Kick off your course with Company Connect Consultancy by following this link: https://shorturl.at/LVq6n
Company Connect Consultancy
+91-9691633901
Very well put. for more information visit us on clinical research training program.