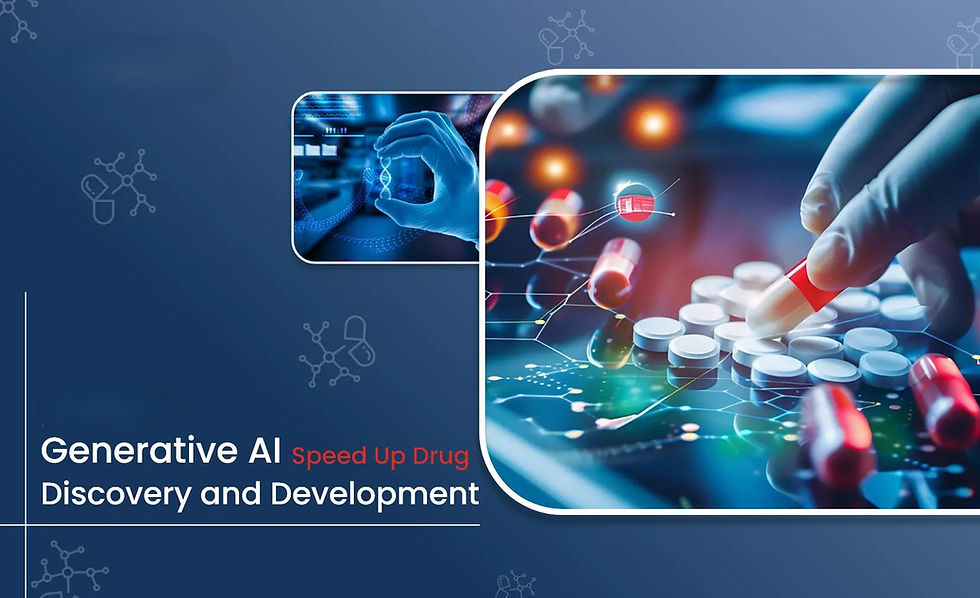
Introduction
The drug discovery and development process is a complex and multi-stage journey that involves the identification of new potential therapeutic targets, the screening of compounds, preclinical testing, and extensive clinical trials before approval for public use. The process begins with basic research, where scientists identify molecular targets that may be responsible for diseases. After this, compounds are tested in labs for their ability to interact with these targets. Promising candidates proceed to preclinical testing, where they are evaluated in animal models for safety and efficacy. If successful, they enter clinical trials in human subjects, progressing through three main phases before receiving regulatory approval. The final phase includes post-marketing surveillance to monitor long-term effects.
Innovation in pharmaceuticals plays a crucial role in improving healthcare outcomes and advancing medical science. The continuous development of novel drug formulations, delivery methods, and therapeutic targets ensures that previously untreatable diseases can be managed or cured. Breakthroughs in areas like personalized medicine, biologics, and gene therapy have revolutionized the treatment of conditions such as cancer, genetic disorders, and autoimmune diseases. Without innovation, medical progress would stagnate, and many diseases would remain without effective treatments.
In recent years, Artificial Intelligence (AI) has increasingly influenced the pharmaceutical industry, revolutionizing drug discovery and development. AI technologies, including machine learning and data analysis algorithms, enable faster identification of drug candidates, optimization of compound properties, and more accurate predictions of clinical trial outcomes. AI can analyze vast datasets, uncover hidden patterns, and streamline the design of drugs, reducing both the time and cost associated with traditional drug development. This technological advancement enhances innovation, making drug discovery more efficient and facilitating the development of drugs tailored to individual genetic profiles.
Kick off your course with Company Connect Consultancy by following this link: https://shorturl.at/LVq6n
The Significance of AI in Drug Discovery
Artificial Intelligence (AI) in pharmaceuticals refers to the use of advanced computational algorithms and machine learning techniques to improve various aspects of drug discovery, development, and production. The primary purpose of AI in this field is to enhance the efficiency, accuracy, and speed of discovering novel drugs, predicting their effectiveness, and optimizing their development processes. AI technologies, such as neural networks, natural language processing, and predictive analytics, enable researchers to make data-driven decisions, thus accelerating drug discovery and reducing the likelihood of failures in clinical trials.
One of the most significant enhancements AI brings to pharmaceuticals is the ability to process and analyze vast datasets quickly and accurately. These datasets include complex biological information, clinical trial results, patient records, and molecular data. By using AI to mine these large volumes of data, researchers can identify potential drug candidates, predict molecular interactions, and uncover hidden insights that were previously difficult to detect through traditional methods. This data-driven approach not only speeds up the research process but also improves the precision of drug development by identifying more promising candidates early on.
AI has also significantly reduced the timeframes for drug discovery. Traditional drug development can take over a decade, but AI-driven techniques streamline many stages of the process. AI can predict the outcomes of early-stage trials, optimize clinical trial design, and identify patient populations more likely to respond to treatment. As a result, drugs are brought to market faster, at a lower cost, and with higher success rates.
Kick off your course with Company Connect Consultancy by following this link: https://shorturl.at/LVq6n
Key Applications of AI in Drug Discovery
Target Identification
Target identification is a critical first step in drug discovery, as it involves identifying molecular targets that play a central role in disease processes. AI algorithms have revolutionized this stage by efficiently analyzing large biological datasets to pinpoint potential drug targets with higher accuracy and speed. These algorithms utilize machine learning techniques, such as deep learning and natural language processing, to analyze genetic, proteomic, and genomic data, as well as scientific literature. By recognizing patterns in the data, AI can identify proteins, genes, or pathways that are associated with specific diseases, thus uncovering new opportunities for therapeutic intervention. Additionally, AI can predict how a target might interact with various drug candidates, streamlining the discovery of highly effective treatments.
Several case studies highlight the successful use of AI in target identification. For example, in cancer research, AI has been employed to analyze vast datasets of patient genomic profiles to identify mutations and genetic alterations that drive cancer development. One prominent case is the use of AI by Insilico Medicine, which employed deep learning algorithms to predict novel drug targets for aging-related diseases, eventually leading to the discovery of potential therapeutic interventions for fibrosis. Similarly, Atomwise, a company specializing in AI-driven drug discovery, used AI to analyze molecular data and identify promising targets for diseases like Ebola and multiple sclerosis. These successful examples underscore the power of AI in transforming the early stages of drug discovery.
Drug Design
In drug design, machine learning plays a pivotal role by enhancing the process of designing novel drug compounds with high efficacy and specificity. Machine learning algorithms analyze vast datasets of molecular structures and biological data to predict which compounds are most likely to interact effectively with a given target. These algorithms are trained to recognize patterns and relationships between chemical structures and their biological activities, enabling the design of molecules that are more likely to succeed in preclinical and clinical trials. Additionally, machine learning can optimize the properties of drug candidates, such as their stability, bioavailability, and toxicity, ensuring that only the most promising compounds move forward in the development pipeline.
AI offers several key advantages in predicting molecular interactions, one of the most critical aspects of drug design. Traditional methods for predicting these interactions can be time-consuming and imprecise, but AI accelerates this process by modeling molecular interactions through computational simulations. Using techniques like molecular docking, deep learning models can predict how small molecules bind to specific protein targets with high accuracy. This ability to predict interactions with precision reduces the need for costly and lengthy experimental trials. AI also enables virtual screening, allowing researchers to quickly assess large libraries of compounds for their potential efficacy, significantly reducing the time and cost associated with experimental screening. The use of AI in drug design not only improves the likelihood of finding successful drug candidates but also facilitates the development of personalized medicines tailored to individual genetic profiles.
Preclinical Testing
Preclinical testing is a crucial phase in drug development that involves assessing the safety, efficacy, and pharmacokinetics of a drug candidate before human trials. AI has dramatically enhanced this stage by simulating and modeling biological systems, offering researchers the ability to predict how drugs will behave in the body without the need for extensive animal testing. AI-driven models, such as computational biology and in silico simulations, can replicate biological processes at the cellular, tissue, and organ levels. These models are trained on vast datasets from genomics, proteomics, and toxicology studies, allowing AI to predict potential drug toxicity, absorption rates, and side effects, thus providing more accurate and reliable preclinical data. AI’s ability to simulate drug interactions with biological systems accelerates the decision-making process, reducing the time spent in preclinical studies.
One of the significant advantages of AI in preclinical testing is the reduction in reliance on traditional experimental methods, such as animal testing, which are costly, time-consuming, and sometimes controversial. By using AI to predict the outcomes of drug interactions and model disease progression, researchers can prioritize the most promising candidates for further testing. AI also allows for high-throughput screening, rapidly analyzing hundreds of potential compounds in a fraction of the time compared to traditional methods. This reduction in reliance on physical experimentation not only lowers costs but also increases the efficiency of the drug development process, enabling faster transitions to clinical trials with more reliable data.
Clinical Trials Optimization
Clinical trials are a critical step in drug development, and optimizing this process can significantly reduce time to market for new treatments. AI plays a pivotal role in speeding up patient recruitment, one of the most challenging and time-consuming aspects of clinical trials. AI analytics can analyze vast datasets, including electronic health records (EHRs), genetic information, and patient demographics, to identify eligible participants who meet the specific criteria for a trial. By using machine learning algorithms to match patients with suitable trials more efficiently, AI helps overcome the traditional barriers of recruitment, such as geographic limitations and slow participant enrollment. This not only accelerates the process but also ensures a more diverse and representative sample of patients for clinical studies.
AI also contributes significantly to predictive modeling for trial outcomes, another crucial component of clinical trial optimization. By analyzing historical trial data, patient responses, and biomarkers, AI can predict how different patient populations will respond to treatments. Predictive modeling enables researchers to identify potential challenges early on, such as patient dropout rates or adverse reactions, allowing for proactive adjustments in trial design. Furthermore, AI-driven models can suggest the most effective dosing regimens, the optimal timing for treatment administration, and the likelihood of a trial’s success. This level of data-driven insight increases the precision of clinical trials, improving their efficiency and reducing the risk of failure.
Kick off your course with Company Connect Consultancy by following this link: https://shorturl.at/LVq6n
Benefits of Implementing AI in Drug Development
Cost Efficiency
AI has become a game-changer in reducing developmental costs in the pharmaceutical industry by streamlining various stages of drug discovery and development. AI algorithms can predict molecular interactions, optimize drug candidates, and simulate biological systems, thus minimizing the need for expensive experimental trials and lengthy preclinical testing. By leveraging AI for tasks such as virtual screening, drug design, and predictive modeling, researchers can focus on the most promising candidates, eliminating the need for time-consuming and costly trial-and-error approaches. Additionally, AI's ability to analyze vast datasets accelerates decision-making, reducing the duration of research and development phases. A prime example of successful cost-saving through AI is Insilico Medicine, which used AI to discover new drug candidates for fibrosis and aging-related diseases, significantly cutting down on research time and costs. AI has also been employed by Atomwise in its drug discovery platform, where it used AI to screen over a billion compounds in just a few days, resulting in faster identification of potential leads and reducing experimental costs.
Enhanced Precision and Accuracy
AI enhances precision and accuracy in the pharmaceutical industry by improving diagnostic capabilities and predicting patient responses more reliably. In diagnostics, AI-driven image recognition and data analysis tools can detect diseases such as cancer at earlier stages by analyzing medical imaging, pathology slides, and genomic data. In drug development, AI helps to predict how individual patients will respond to treatments based on their genetic makeup and other biomarkers, leading to more personalized and effective therapies. By integrating real-world data from clinical practice, electronic health records (EHRs), and patient-reported outcomes, AI refines the drug development process, ensuring that treatments are optimized for specific patient populations. For instance, IBM Watson Health has worked with various healthcare providers to integrate AI-driven insights from real-world data, improving treatment accuracy for cancer patients. This integration enhances drug development by identifying more precise biomarkers and refining clinical trial designs.
Accelerated Time-to-Market
AI plays a critical role in accelerating the time-to-market for drugs by streamlining processes from lab to market. AI tools are used throughout the entire drug development pipeline, from initial target identification and compound screening to clinical trial optimization and post-market surveillance. By automating data analysis, optimizing trial designs, and predicting clinical trial outcomes, AI reduces the time required to bring a drug from the lab to the market. For example, AI-powered platforms like those used by companies such as BenevolentAI have shortened the timeline for drug discovery by analyzing complex biological data to identify new therapeutic targets. Additionally, DeepMind's AI was instrumental in rapidly developing insights into the structure of the SARS-CoV-2 virus, facilitating the accelerated development of COVID-19 vaccines. These cases demonstrate how AI has enabled the pharmaceutical industry to reduce development timelines, resulting in faster access to life-saving treatments.
Kick off your course with Company Connect Consultancy by following this link: https://shorturl.at/LVq6n
Challenges and Limitations of AI in Drug Development
Data Quality and Availability
High-quality data is crucial for the effectiveness of AI in drug discovery and development. AI algorithms rely on large, diverse datasets to train models that can predict drug efficacy, patient responses, and potential safety concerns. Without accurate, comprehensive, and well-curated data, the results generated by AI models can be unreliable or even misleading. Data sources like electronic health records (EHRs), genomic data, and clinical trial results must be high in quality to ensure that AI systems can make informed predictions. However, challenges with data sharing and privacy can hinder the availability of such data. Regulations such as the Health Insurance Portability and Accountability Act (HIPAA) in the U.S. and the General Data Protection Regulation (GDPR) in the EU place strict constraints on the sharing of personal health data, making it difficult to pool large datasets for training AI systems. Balancing data privacy with the need for high-quality, accessible data remains a significant challenge.
Regulatory Considerations
The use of AI in drug discovery is subject to various regulations to ensure that the technology is used safely and effectively. Regulatory bodies like the U.S. Food and Drug Administration (FDA) and the European Medicines Agency (EMA) have started to establish frameworks for the use of AI in clinical trials, data analysis, and drug development. These frameworks often include guidelines for transparency in AI model development, data validation, and ensuring that AI systems adhere to existing standards for drug safety and efficacy. However, as AI technology continues to evolve, the regulatory landscape is still catching up. In the future, we can expect more detailed and specialized regulations to address issues such as algorithmic accountability, the validation of AI-driven findings, and ensuring that AI technologies do not exacerbate health disparities. Regulatory considerations will also need to ensure that AI systems are interpretable and can provide clear, explainable reasoning for their decisions in the drug development process.
Ethical Considerations
AI in drug testing and patient safety raises several ethical concerns, particularly regarding fairness, transparency, and accountability. The use of AI in drug discovery, clinical trials, and patient monitoring introduces the potential for bias if algorithms are trained on incomplete or non-representative datasets, which could disproportionately impact certain populations. Additionally, the opacity of many AI models—especially deep learning systems—raises concerns about their decision-making processes. If AI algorithms cannot provide clear explanations for their recommendations, it could lead to a lack of trust in AI-driven decisions, especially when patient safety is at stake. Therefore, ensuring transparency and accountability in AI systems is paramount, particularly in critical areas like drug safety and patient outcomes. Ethical considerations also include ensuring that AI does not replace the need for human oversight, especially in areas that directly affect patient well-being. The development of AI should be governed by ethical frameworks that prioritize patient safety, fairness, and transparency.
Kick off your course with Company Connect Consultancy by following this link: https://shorturl.at/LVq6n
Future Prospects of AI in Drug Discovery
The future of AI in pharmaceuticals holds immense promise, with predictions pointing toward significant breakthroughs that will revolutionize the industry. As AI technology continues to advance, we can expect increasingly sophisticated algorithms capable of more accurate predictions in drug discovery, personalized medicine, and treatment outcomes. AI will likely play a central role in predicting rare diseases, identifying novel drug targets, and designing drugs that are tailored to individual genetic profiles. Furthermore, AI-driven systems will become more integrated into real-time diagnostics and patient monitoring, enabling proactive and precision-based healthcare. Machine learning and deep learning models will become even more adept at interpreting complex biological data, potentially leading to faster identification of therapeutic opportunities and more efficient drug development pipelines.
Potential breakthroughs on the horizon include the development of AI-powered drug discovery platforms that can design drugs faster, more efficiently, and at a lower cost. Innovations in areas like gene editing, such as CRISPR technology, could be accelerated through AI, leading to groundbreaking therapies for genetic diseases. AI could also help in creating highly personalized treatments that are tailored to an individual’s genetic makeup and health history, moving toward the era of precision medicine. Another exciting prospect is the integration of AI with blockchain technology to improve data integrity and transparency in clinical trials, further enhancing the reliability of research.
However, these advancements will require close collaboration between AI experts and pharmaceutical scientists. AI's potential in drug discovery and development is maximized when paired with the deep domain knowledge of scientists who understand the intricacies of biology, chemistry, and medicine. By working together, AI specialists and pharmaceutical researchers can ensure that AI is applied effectively and ethically, leading to better drug candidates and improved patient outcomes. Collaboration is key to overcoming challenges such as data sharing, regulatory compliance, and ethical considerations while driving forward the next generation of breakthroughs.
Kick off your course with Company Connect Consultancy by following this link: https://shorturl.at/LVq6n
Conclusion
AI has already had a transformative impact on drug discovery and development, significantly accelerating processes and improving outcomes across various stages. From target identification to clinical trials, AI technologies such as machine learning, deep learning, and predictive analytics have streamlined traditionally time-consuming and costly procedures. AI's ability to analyze vast datasets, predict molecular interactions, and simulate biological systems has led to faster identification of drug candidates, reduced development costs, and improved precision in targeting diseases. Its role in optimizing patient recruitment, enhancing trial designs, and predicting treatment responses has further revolutionized how new therapies are brought to market. AI's ability to integrate real-world data and improve diagnostics also paves the way for more personalized and efficient treatments.
The potential for AI to revolutionize healthcare is immense. As AI continues to evolve, it promises to unlock new frontiers in precision medicine, where treatments are tailored to the individual genetic makeup and health conditions of patients. With AI’s capabilities in predictive modeling, it could reduce healthcare disparities, improve patient outcomes, and even aid in the discovery of cures for currently untreatable diseases. By reducing costs, improving drug efficacy, and accelerating development timelines, AI has the power to make life-saving therapies more accessible and timely. However, the success of AI in healthcare will depend on continued collaboration between AI experts, pharmaceutical scientists, regulatory bodies, and ethical guidelines to ensure responsible, transparent, and equitable use of these technologies.
Kick off your course with Company Connect Consultancy by following this link: https://shorturl.at/LVq6n
Company Connect Consultancy
+91-9691633901
Comments